The True Cost of AI: Balancing Security, Scalability, and ROI
- Cameron Duncan
- 2 days ago
- 5 min read
Introduction
The excitement around artificial intelligence is palpable. Businesses everywhere feel the pressure to innovate, to harness the power of AI, and to keep pace with competitors who are already integrating these tools into their operations.
But as organizations rush to adopt AI, it’s easy to focus on the obvious costs - licensing fees, subscriptions, or application programing interface (API) usage - while overlooking the bigger picture. The real cost of AI is about much more than what you pay up front. It’s about finding the right balance between security, scalability, and return on investment (ROI).
The Options for AI Deployment
When it comes to deploying AI, organizations have three main options: fully on-premises, private cloud, and public SaaS (Software as a Service).
Fully On-Premises:
This option involves hosting large language models and all supporting infrastructure within your own data center. It requires significant investment in hardware (like GPUs and servers), energy, and ongoing maintenance. You also need specialized talent to manage, update, and secure these systems.
Private Cloud:
Here, the AI solution is hosted in a cloud environment that is dedicated to your organization. You retain control over your data and infrastructure, but still benefit from the scalability and flexibility of the cloud.
Public SaaS:
The most common approach, public SaaS, means using AI tools hosted by a third-party provider. This is the easiest to get started with, as it requires no infrastructure investment and minimal technical expertise.
When it comes to deploying AI, organizations have three main options: fully on-premises, private cloud, and public SaaS (Software as a Service).
Each option comes with its own technical and financial requirements. On-premises and private cloud deployments demand more up-front investment and operational complexity, while public SaaS offers convenience at the cost of control and, sometimes, security.
The Costs of On-Premises AI
The idea of hosting AI entirely on-premises is appealing for those who want maximum control over their data and processes. However, the hidden costs are substantial. Setting up the necessary infrastructure means purchasing expensive, power-hungry GPUs and servers often costing between $100,000 and several million dollars just to achieve the speed and performance modern AI tools require. Beyond hardware, there’s the ongoing expense of energy, cooling, and physical space.

But the costs don’t stop there. Running on-premises AI demands a team of specialized talent to manage, secure, and continually improve the system. Process improvement becomes an ongoing project, not a one-time setup. For most organizations, this level of investment only makes sense at massive scale, where the ROI can justify the outlay. For everyone else, the up-front and ongoing costs are simply prohibitive.
The Hidden Tradeoffs of Multi-Tenant SaaS AI
Public SaaS AI platforms are popular for their ease of use and rapid deployment, requiring minimal infrastructure investment and technical expertise. However, they operate on a multi-tenant architecture where data from multiple customers is stored together in shared environments, increasing the risk of accidental exposure or unauthorized access.
While public SaaS makes it easy to get started with AI, this convenience comes at the cost of reduced control over data governance and security.
Real-world incidents like data deletions and misconfigurations highlight the vulnerabilities inherent in these setups. Organizations also have limited visibility into who accesses their data and how it’s used, relying heavily on the provider’s internal controls, which can be compromised by insider threats or human error. These centralized platforms are attractive targets for cyberattacks, where a single vulnerability can expose thousands of customers’ data.
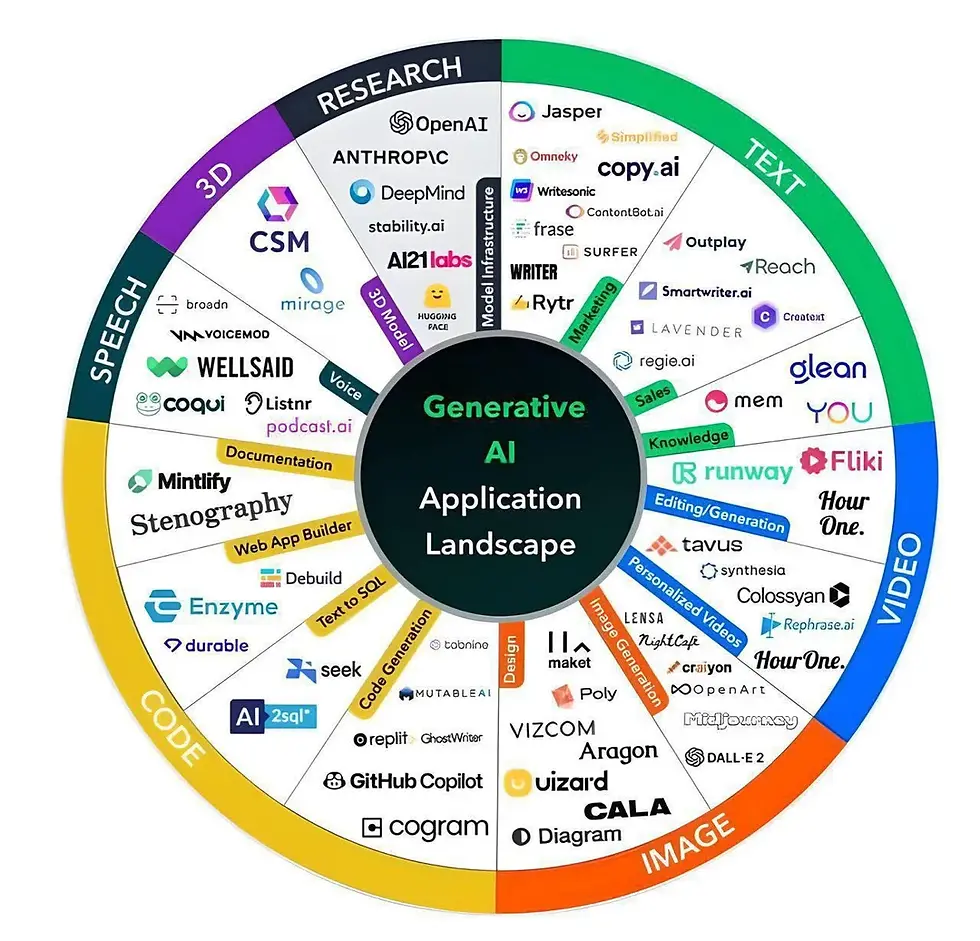
Additionally, public SaaS solutions often create data silos that fragment information across multiple platforms, complicating data integration and compliance efforts. While public SaaS makes it easy to get started with AI, this convenience comes at the cost of reduced control over data governance and security.
The Economics of Cloud-Based AI
Cloud-based AI, especially through APIs, offers a compelling alternative. Instead of investing heavily in hardware and infrastructure, organizations can access powerful AI models on a pay-as-you-go basis. This dramatically reduces up-front costs and allows businesses to scale their usage up or down as needed.
Privately hosted solutions in your own cloud environment offer a middle ground. You get the cost efficiency and scalability of the cloud, but retain control over your data, access, and security policies.
The flexibility of cloud APIs means you only pay for what you use. Token pricing (charging by the amount of data processed or the number of requests) makes it easy to align costs with actual business needs. Compared to the capital and operational expenses of on-premises deployments, cloud-based AI is often measured in pennies per transaction, making it accessible to organizations of all sizes.
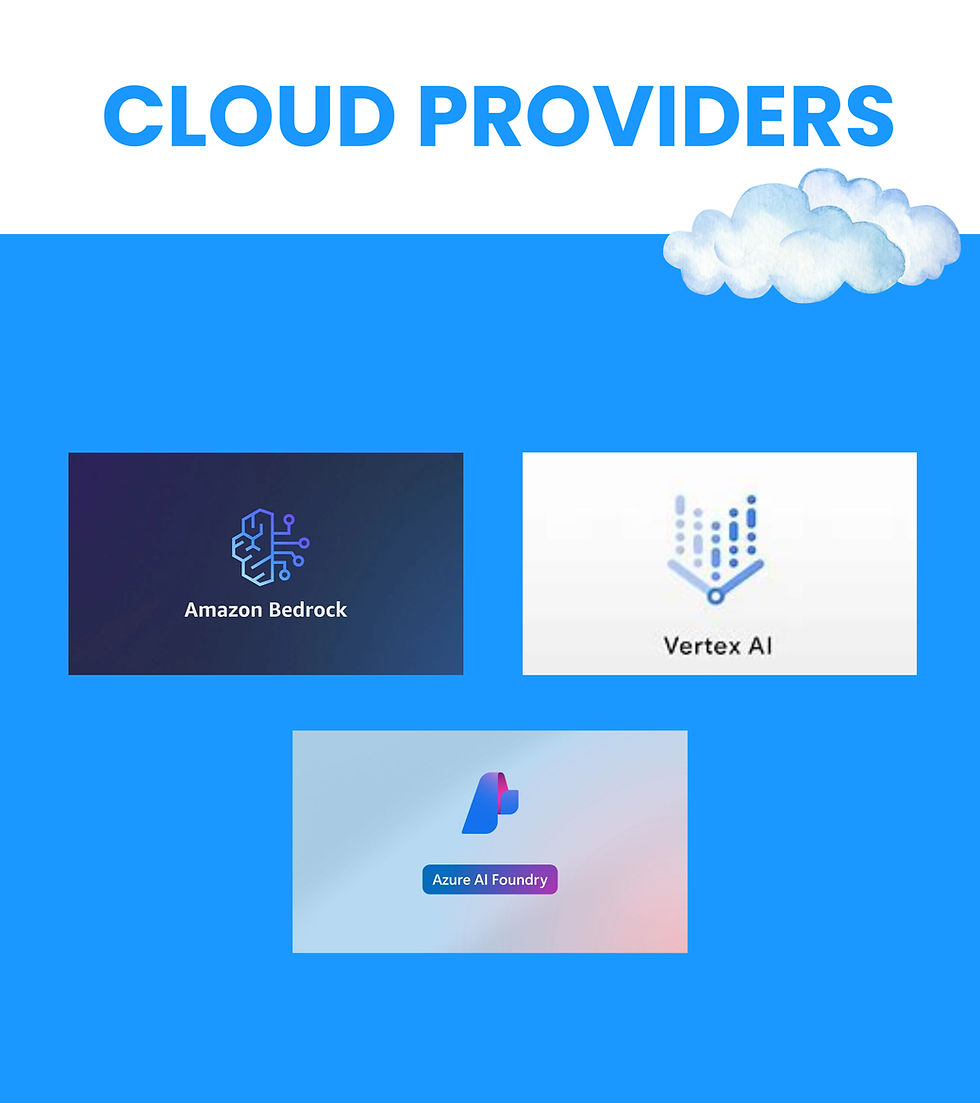
Scalability is another major advantage. If your business needs change, you can quickly adjust your usage without worrying about sunk costs in hardware or the need to hire additional staff. This agility is crucial in a rapidly evolving AI landscape.
Achieving ROI with Secure, Cost-Effective AI
The best ROI comes from solutions that strike the right balance between security and cost. While public SaaS is convenient, it often means giving up control over your data. This can be a dealbreaker for organizations with sensitive information or strict compliance requirements.
Privately hosted solutions in your own cloud environment offer a middle ground. You get the cost efficiency and scalability of the cloud, but retain control over your data, access, and security policies. By architecting your AI deployment so that you control your own API keys and cloud instances, you minimize third-party risk while maximizing flexibility.
To truly realize the value of AI, it’s not enough to simply deploy the technology. You need to integrate it into your business processes, ensuring that it drives real improvements in efficiency, decision-making, and customer experience. The payback on these tools is measured in months, not years, provided you choose a model that aligns with your organization’s needs and risk tolerance.
To truly realize the value of AI, it’s not enough to simply deploy the technology. You need to integrate it into your business processes, ensuring that it drives real improvements.
Conclusion
The smartest AI investments are those that align with both your security requirements and your business goals. Don’t be fooled by low up-front costs or the promise of instant scalability. Look at the total cost of ownership, the long-term ROI, and the level of control you need over your data.
Evaluate your AI strategy with a critical eye. Consider not just what you’re paying today, but what you might pay tomorrow in terms of risk, compliance, and missed opportunities. The right balance of security, scalability, and ROI will set your organization up for sustainable success in the age of AI.
If you’re ready to take a closer look at your AI plans and want a clear, unbiased cost-benefit analysis, reach out for a consultation. Make sure your next AI investment is the right one for your business.